Semantics and knowledge graphs can be leveraged to feed data to recommendation systems, enhancing their accuracy and effectiveness.
Recommendation engines in today’s world require a vast number of relationships to make effective recommendations. Using semantics and knowledge graphs enable:
Entity-based Recommendations:
Knowledge graphs capture semantic relationships between entities such as products, items, users, and their attributes. By integrating the knowledge graph into the recommendation system, it becomes possible to recommend items based on their semantic similarity. For example, if a user is interested in a particular product, the recommendation system can leverage the knowledge graph to identify related or similar items and suggest them to the user.
Contextual Recommendations:
Semantics and knowledge graphs enable the incorporation of contextual information into recommendation systems. The knowledge graph can represent various contextual factors such as time, location, user preferences, or historical behavior. By considering these contextual attributes and their relationships, recommendation systems can provide more personalized and context-aware recommendations. For instance, recommending restaurants based on a user’s location, time of day, and cuisine preferences.
Serendipitous Recommendations:
Knowledge graphs can facilitate serendipitous recommendations by leveraging the connections between seemingly unrelated items. The knowledge graph can identify items that have indirect or lesser-known connections to a user’s preferences. This allows recommendation systems to suggest diverse and unexpected recommendations, introducing users to new and potentially interesting items they may not have discovered otherwise.
Hybrid Recommendation Approaches:
Semantics and knowledge graphs can be combined with other recommendation techniques, such as collaborative filtering or content-based filtering, to create hybrid recommendation models. The knowledge graph can serve as an additional source of information to enhance the accuracy and coverage of recommendations. It can complement user behavior data and item attributes, providing a more comprehensive understanding of user preferences and item relationships.
Cold-start Problem Mitigation:
The cold-start problem refers to situations where there is limited or no data available for new users or items. Semantics and knowledge graphs can help mitigate this problem by providing a foundation for making recommendations even in the absence of user-specific data. By leveraging the relationships and attributes captured in the knowledge graph, recommendation systems can make intelligent inferences and suggest relevant items to new users or items based on their semantic characteristics.
Cross-Domain Recommendations:
Knowledge graphs can facilitate recommendations across different domains or categories. By capturing the relationships between entities from various domains, recommendation systems can leverage the knowledge graph to suggest items that are relevant in one domain based on a user’s preferences in another domain. This enables cross-domain recommendations, expanding the scope of recommendations and providing users with diverse and personalized suggestions.
Explainable Recommendations:
Semantics and knowledge graphs can contribute to explainable recommendations, allowing users to understand the reasoning behind the recommendations. The knowledge graph can provide transparency by showcasing the relationships and attributes that influenced the recommendation. This helps build trust with users and allows them to have a clearer understanding of why specific items were suggested to them.
Related Knowledge
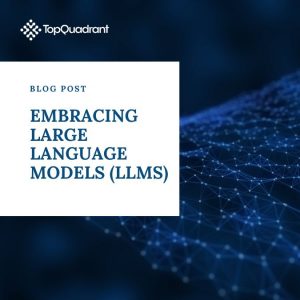
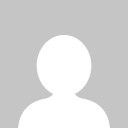
How TopQuadrant is embracing Large Language Models (LLMs)
Resource Hub Search Table of Contents < All Topics Main Data Governance How TopQuadrant is embracing Large Language Models (LLMs) Print How TopQuadrant is embracing
Enhancing Data Interoperability in Life Sciences
Resource Hub Search Table of Contents < All Topics Main Data Governance Enhancing Data Interoperability in Life Sciences Print Enhancing Data Interoperability in Life Sciences
The Road to AI Success: Avoiding Common Pitfalls in Enterprise AI Pilots
Resource Hub Search Table of Contents < All Topics Main Data Governance The Road to AI Success: Avoiding Common Pitfalls in Enterprise AI Pilots Print
Semantic Search with Knowledge Graphs
Traditional keyword-based search engines fall short in delivering relevant results. Semantic search with knowledge graphs is the answer.
Leveraging Semantics to Enable Zero-Trust Architectures in Government Systems
Learn how government agencies can establish a Zero-Trust model while complying with open data requirements in this white paper.
How can Semantic Information Management Help to Preserve Meaning in a Dynamic Data Environment?
By connecting all kinds of data, semantic data management systems empower users to unlock the true meaning behind their business’s data.